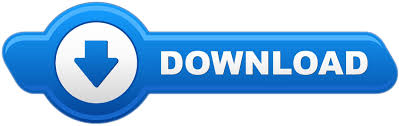
7.2.8 - Simultaneous (1 - α) x 100% Confidence Intervals.7.2.7 - Testing for Equality of Mean Vectors when \(Σ_1 ≠ Σ_2\).7.2.6 - Model Assumptions and Diagnostics Assumptions.7.2.4 - Bonferroni Corrected (1 - α) x 100% Confidence Intervals.7.2.2 - Upon Which Variable do the Swiss Bank Notes Differ? - Two Sample Mean Problem.7.2.1 - Profile Analysis for One Sample Hotelling's T-Square.7.1.15 - The Two-Sample Hotelling's T-Square Test Statistic.7.1.12 - Two-Sample Hotelling's T-Square.7.1.11 - Question 2: Matching Perceptions.7.1.8 - Multivariate Paired Hotelling's T-Square.7.1.7 - Question 1: The Univariate Case.7.1.4 - Example: Women’s Survey Data and Associated Confidence Intervals.7.1.1 - An Application of One-Sample Hotelling’s T-Square.Lesson 7: Inferences Regarding Multivariate Population Mean.6.2 - Example: Wechsler Adult Intelligence Scale.Lesson 6: Multivariate Conditional Distribution and Partial Correlation.5.2 - Interval Estimate of Population Mean.5.1 - Distribution of Sample Mean Vector.Lesson 5: Sample Mean Vector and Sample Correlation and Related Inference Problems.4.7 - Example: Wechsler Adult Intelligence Scale.4.6 - Geometry of the Multivariate Normal Distribution.4.4 - Multivariate Normality and Outliers.4.3 - Exponent of Multivariate Normal Distribution.Lesson 4: Multivariate Normal Distribution.Lesson 3: Graphical Display of Multivariate Data.Lesson 2: Linear Combinations of Random Variables.1.5 - Additional Measures of Dispersion.

Lesson 1: Measures of Central Tendency, Dispersion and Association.
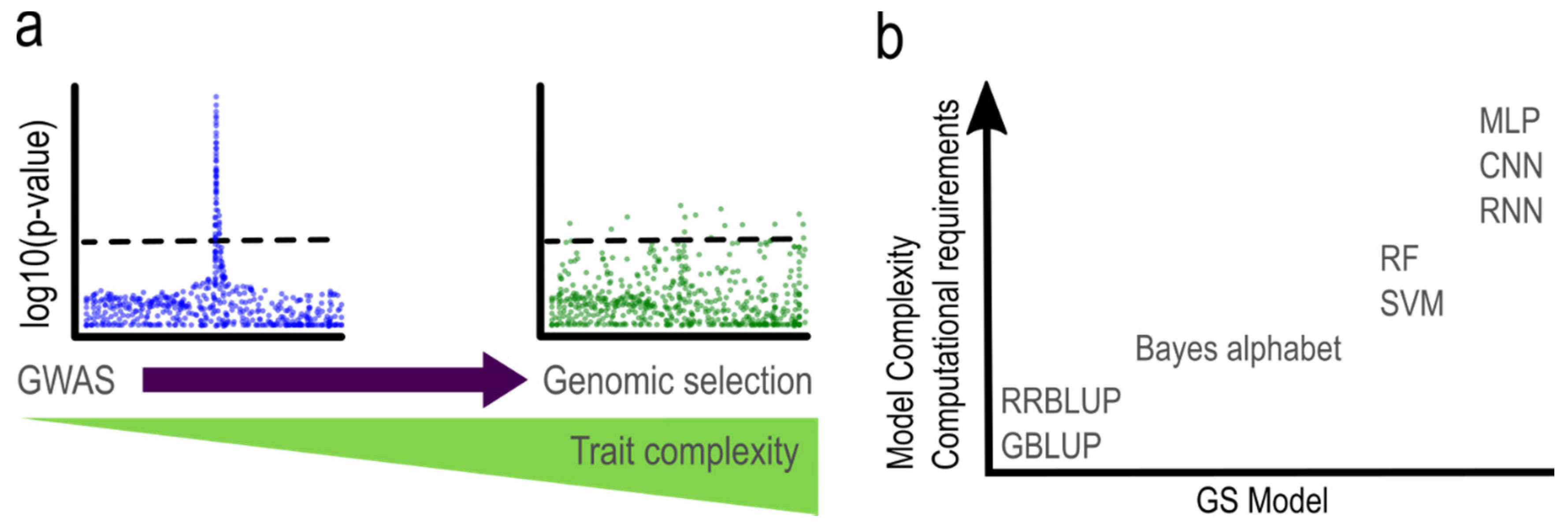
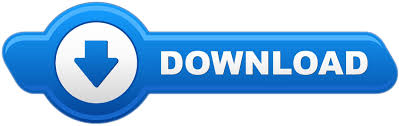